Sales Analytics Hub
Empower your sales organization with insights generated by Generative AI, sourced from a centralized data hub.
Streamline Sales Analytics: Get Answers Faster with Conversational AI
Central sales analytics teams handle a substantial amount of data and analytical projects aimed at providing a deeper understanding of sales performance.
Frequently, the sales department has numerous unanswered queries due to constrained resources and high demand faced by these teams.
By leveraging Generative AI, particularly large language models (LLMs), along with Conversya, sales leaders can adopt a straightforward conversational method to derive valuable analyses using a shared data foundation.
Key Features
Enable Data-Driven Decisions
Equip sales leaders to promptly tackle challenges with reliable data and effortlessly conduct personalized analysis.
Boost Capability
Empower central sales analytics teams to prioritize impactful insights over addressing ad-hoc inquiries.
Enhance Collaboration
Accelerate response times and communication among teams.
Enhance Overall Visibility
Make data more accessible for a better understanding of the current pipeline status at any time.
How It Works
Within Conversya, a sales analytics initiative has been established. This initiative connects to a user-friendly self-service analytics exploration application designed for sales professionals.
They can effortlessly obtain answers by entering simple requests, such as:
-
Provide an overview of sales for a specific store over the past three months.
-
Rank the sales for a particular product across all stores.
-
Identify the stores with the best performance.
-
Display sales data for a chosen product over the last year.
These prompts, along with data schema and potential small data samples, are conveyed to a Large Language Model (LLM) through an API. Sales leaders leverage the generated answers to swiftly address queries.
The model's responses are drawn from the entire dataset, ensuring ongoing relevance and broad scope, regardless of data volume.
To manage data sensitivity, the public version of the API can be utilized. Alternatively, a containerized version of the LLM provides more rigorous control over data and input.
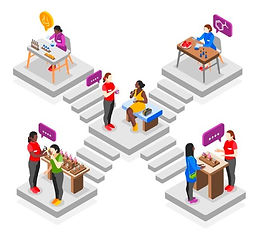
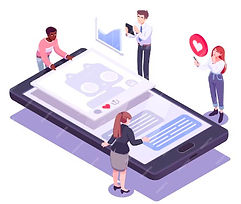


Accountability Considerations
Apart from an overarching Responsible AI policy to ensure uniform practices across AI initiatives, specific recommendations for this case include:
Transparency and User Awareness
Clearly mark AI-generated insights and clarify user interaction with an AI system. Document model limitations and encourage users to exercise prudent judgment when working with model outputs, especially in cases involving potential human safety concerns.
Monitoring and Error Management
Closely monitor the underlying model's error rate, particularly in critical scenarios. Provide a panel to enhance transparency, offering users visibility into the columns and datasets contributing to the insights.
Training and Scope Definition
Train teams to define the usage scope, given the real-time nature of responses and the variability of exact responses based on data and LLM behavior. Retroactive auditability isn't supported, so clear guidelines and training are essential.