Transform Customer Reviews into Actionable Insights
Dive deeper than surface-level patterns and trends from customer reviews using Generative AI, particularly large language models (LLMs).
Apply this technology to extract elements from reviews, encompassing entity recognition metadata and conducting sentiment analysis on precise product aspects like size, fit, quality, fabric, color, and other specific attributes.
Within this Conversya-built use case, users can obtain these insights via a self-service analytics generation application, provided the input is validated and integrated into a structured dataset.
Feature Highlights
Unearth Patterns & Anticipate Challenges
Effortlessly explore extensive data sets with straightforward queries to uncover insights that were once inaccessible. Identify surges in complaints related to specific product features, enabling proactive corrective measures.
Enhance Collaboration and Streamline Operations
Empower diverse teams along the integrated value chain to pose relevant queries tailored to their roles. Foster collaboration among marketing, product development, supply chain, and sales.
Mitigate Data-Related Risks
Extract personal data from reviews to reduce data governance demands.
How It Works
An initial LLM scrutinizes the reviews and autonomously extracts pertinent information in a structured format. The tool offers a user-friendly interface that validates the extracted data by highlighting specific text segments for each category.
This enables product or customer analysts to review and edit the text if necessary before saving it as structured data.
A self-service analytics application utilizes a second LLM to furnish responses and insights for any user query. Upon receiving a query, the model generates a series of Conversya instructions executed locally to create the required dashboard, presenting the user with a personalized response to their inquiry.
This method ensures the model maintains consistent relevance and broad applicability, regardless of the scale or intricacy of the underlying data, while upholding the utmost level of data privacy.
No actual data values are transmitted to the model during the process, ensuring stringent data security.
Accountability Considerations
Alongside an encompassing Responsible AI policy that maintains uniform practices throughout AI projects:
Ensure Data Privacy Protection
Implement robust security measures to safeguard model interactions and protect data privacy.
Human Review and Feedback
Enable human reviewers to provide feedback on model performance, enhancing the underlying extraction algorithm.
Regular Output Review
Consistently review output data to prevent the extraction of private or personal information by the model.
Bias Prevention and Accuracy
Have data scientists regularly assess the accuracy of extraction data, ensuring consistency and fairness across various subgroups. This guarantees that no biases influence the prioritization of certain information during extraction.

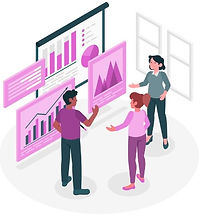
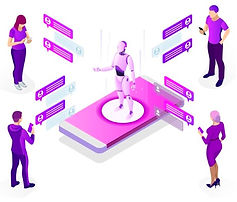
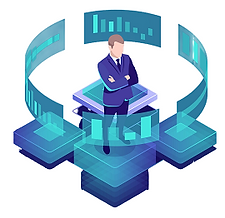